There is a need to make climate science more agile and more responsive, and that means moving (some of it) from research to operations.
Readers here will know that the climate science community has had a hard time giving quantitative explanations for what’s happened in climate over the last couple of decades. Similarly, we are still using scenarios that were designed more than a decade ago and have not been updated to take account of the myriad changes that have happened since. Many people have noticed these problems and so there are many ideas floating around to fix them.
As someone who works in one of the main modeling groups that provide their output to the IPCC and NCA assessments, and whose models inform the downscaled projections that are used in a lot of climate resilience work, I’ve been active in trying to remedy this state of affairs. For the CERESMIP project (Schmidt et al., 2023) we proposed updating the forcings datasets and redoing much of the attribution work that had been done before to focus specifically on explaining the trends in the CERES time period (2003 to present).
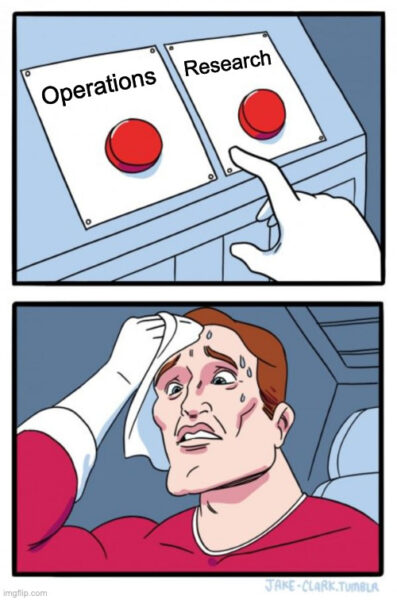
And in this week’s New York Times, Zeke Hausfather and I have an opinion piece arguing that climate science more broadly – and the CMIP process specifically – needs to become more operational. To be clear, this is not a radical notion, nor is it a fringe idea that only we are thinking about. For example, there was a workshop last month in the UK, where discussion of the inputs for the next round of CMIP simulations (CMIP7 for those keeping count) included a lot of discussion about what a ‘sustained’ [footnote1] mode of extensions and updates to the input datasets would look like (and it’s definitely worth scrolling through some of the talks). Others have recently argued for a separate set of new institutions to run operational climate services (Jakob et al, 2023; Stevens, 2024).
Our opinion piece though was very focused on one key aspect – the updating of forcing data files, and the standardization of historical extension simulations by the modeling groups. This has come to the forefront partly because of the difficulties we have had as a community in explaining recent temperature anomalies, and partly as a response to the widespread frustration with the slow pace at which the scenarios and projections are being updated (e.g. Hausfather and Peters (2021)). Both of these issues stem from the realization that climate change is no longer purely a long-term issue for which an assessment updated every decade is sufficient.
The End of History
A big part of the effort to both understand past climate and project future climate is supported by the CMIP program. This is a bottom-up, basically self-organized, effort from the modeling groups to coordinate on what kinds of experiments to run with their models, what kind of data to output, and how one should document these efforts. Since its debut in the early 1990s, this process has become more complex as models have become more complex and the range of useful questions that can be asked of the models has broadened. Where, at the beginning, there was really only one input parameter (the CO2 concentration) that needed to be coordinated, the inputs have now broadened to include myriad forcings related to other greenhouse gases, air pollution, land surface change, ozone, the sun, volcanoes, irrigation, meltwater etc.
Since CMIP3, one of the key sets of experiments has been the ‘historical’ simulations (and various variations on that theme). These are by far the most downloaded datasets and are used by thousands of researchers to evaluate the models over the instrumental period (starting in 1850). But when does ‘history’ end? [footnote2]
In modeling practice, ‘history’ stops a few years before the simulations need to start to impact the IPCC reports. So for 2007 report, the CMIP3 simulations were carried out around 2003, and so history stopped at the end of 2000. For CMIP5, history stopped in 2005, and for CMIP6 (the last go-around), it stopped in 2014. You will note that this is a decade ago.
Forcing the Issue
Depending on the specific forcing, the observations that go into the forcing datasets are available are with different latencies. For instance, sea surface temperatures are available basically in real time, solar irradiance is available after a few days, greenhouse gases a few weeks etc. However, aerosol emissions are not directly observed, but rather are estimated based on economic data that often doesn’t get released for months. Other forcings, like the irrigation data or other land use changes can take years to process and update. In practice, the main bottleneck is the estimate of the emissions of short lived climate forcings (reactive gases, aerosols, etc.), which include things like the emissions from marine shipping. Changes in the other long latency forcings aren’t really expected to have noticeable impacts on an annual or sub-decadal time-scales.
One perennial issue is also worth noting here; over the ~170 years of the historical records there are almost no totally consistent datasets. As instrumentation improved, coverage improved, and when satellite records started to be used, there are changes to the precision, variance, and bias over time. This can partially be corrected for, but for some models, for instance, the switch from decadal averages of biomass burning in the past to monthly varying data in recent years led to quite substantial increases in impacts (since the model’s response was highly non-linear) Fasullo et al., 2022.
Partially in response to this inhomogeneity over time, many of these forcings are in part modeled. For instance, solar irradiance is only directly measured after 1979, and before that has to be inferred from proxy information like sunspot activity. So not only do forcing datasets have to be extended with new data as time passes, but they frequently revise past estimates based on changes to the source data estimates or updates in the modeling. Often the groups do the extension and the update at the same time, which means that the dataset is not continuous with what had been used in the last set of simulations, making hard to do extensions without going back to the beginning.
How far does it go?
One thing that has only become apparent to me in recent months (and this is true for many in the CMIP community), is how widely used the CMIP forcing data has become far outside the original purpose. It turns out that building consistent long term syntheses of climate drivers is a useful activity. For instance, both the ECMWF reanalysis (ERA5) and the MERRA2 effort used the CMIP5 forcings from 2008 onwards for their solar forcing. But these fields are the predictions made around 2004 and are now about half a solar cycle out of sync with the real world. Similarly, the aerosol fields in the UKMO decadal prediction system are from a simulation of 2016 and are assumed fixed going forward. Having updated historical data and consistent forecasts might be key in reducing forecast errors beyond the sub-seasonal timescale.
What can be done?
As we mentioned in the opinion piece, and as (I think) was agreed as a target at the recent workshop, it should be possible to get a zeroth order estimate of the last year’s data by July the following year. i.e. we should be able to get the 2024 data extension by July 2025. That is sufficient for modeling groups to be able to quickly add a year to the historical ensembles and the single forcing/grouped forcing simulations that we use for attribution studies, and for these to be analyzed in time for the WMO State of the Climate report which comes out each November.
If additionally, these extensions can be used to seed short term forecasts (say covering the next five years), they would also be usable for the initialized decadal predictions which are also started in November. Reanalyses could also make use of these short term forecasts to allow for updates in their forcing fields and help those efforts be more realistic.
Of course, the big work right now is to update and extend the historical data from 2014 to at least 2022 or, ideally, 2023, and this should be done very shortly (preliminary versions very soon, finalized versions in the new year). And given these new updated pipelines, building a consensus to extend them on an annual basis should be easier to build.
This will require a matching commitment from the climate modeling groups to do the extensions, process them and upload them to the data in a timely manner, but this is a relatively small ask compared to what they generally do for CMIP as a whole.
As John Kennedy noted recently, we need to shift more generally away from thinking about papers as the way to update our knowledge, to thinking about operational systems that automatically update (as much as possible) and that are continually available for analysis. We’ve now got used to this for surface temperatures and assorted data streams, but it needs to be more prevalent. This would make the attribution of anomalies such as we had in 2023/2024 much easier, and would reveal far more quickly whether there is something missing in our models.
Notes
[footnote1] For some reason, the word “operational” gives some program managers and agencies hives. I think this relates to a notion that making something operational is perceived as being an open-ended commitment that reduces their future autonomy in allocating funding. However, we are constantly being exhorted to do work that is R2O (‘research to operations’), but generally speaking this is assumed to be a hand-off to an existing operational program, rather than the creation of a new one. So ‘sustained’ it is.
[footnote2] Not in 1992, despite popular beliefs at the time.
References
- G.A. Schmidt, T. Andrews, S.E. Bauer, P.J. Durack, N.G. Loeb, V. Ramaswamy, N.P. Arnold, M.G. Bosilovich, J. Cole, L.W. Horowitz, G.C. Johnson, J.M. Lyman, B. Medeiros, T. Michibata, D. Olonscheck, D. Paynter, S.P. Raghuraman, M. Schulz, D. Takasuka, V. Tallapragada, P.C. Taylor, and T. Ziehn, "CERESMIP: a climate modeling protocol to investigate recent trends in the Earth's Energy Imbalance", Frontiers in Climate, vol. 5, 2023. http://dx.doi.org/10.3389/fclim.2023.1202161
- C. Jakob, A. Gettelman, and A. Pitman, "The need to operationalize climate modelling", Nature Climate Change, vol. 13, pp. 1158-1160, 2023. http://dx.doi.org/10.1038/s41558-023-01849-4
- B. Stevens, "A Perspective on the Future of CMIP", AGU Advances, vol. 5, 2024. http://dx.doi.org/10.1029/2023AV001086
- Z. Hausfather, and G.P. Peters, "RCP8.5 is a problematic scenario for near-term emissions", Proceedings of the National Academy of Sciences, vol. 117, pp. 27791-27792, 2020. http://dx.doi.org/10.1073/pnas.2017124117
- J.T. Fasullo, J. Lamarque, C. Hannay, N. Rosenbloom, S. Tilmes, P. DeRepentigny, A. Jahn, and C. Deser, "Spurious Late Historical‐Era Warming in CESM2 Driven by Prescribed Biomass Burning Emissions", Geophysical Research Letters, vol. 49, 2022. http://dx.doi.org/10.1029/2021GL097420
Good luck. In business, if you push hard enough to get data, you will get something on time. It won’t always be data.
Sincere wishes for success in this.
Another reason for ensuring that models are right up to date, is that climate science will be coming under increasing attack from its opponents over the short term. Individuals connected with the incoming US administration have suggested that they will disband NOAA and the EPA. At the very least, political appointments to the heads of those organisations will cripple their climate related activities. Meanwhile science deniers will seize on any lack of consistency in the models, or any failure to update them, to discredit climate research. I am afraid we are heading into an increasingly toxic political interference in climate science, and active researchers will need to become better at communicating their findings quickly and articulately, as well as defending them against the attacks of malign actors whose expertise lies in generating persuasive alternative narratives.
Rory, do you have any relevant background or are you simply posing as scientist? I presented at NASA a few months ago. There were many from NOAA there as well. I didn’t see you there. I was very critical of everything Gavin’s GISS produces for public consumption,. from ozone to CO2, to warming. Among other things I shared data showing CO2 is mostly from ocean upwelling and that this equatorial ocean upwelling is Solar forced.
They didn’t throw me out, even after I shared a slide revealing that NASA eliminated ocean upwelling from their CO2 model.
and again, I didn’t see you there. Oh, and I’ve lost relatives to Holocaust, you Poser, but thanks for sharing your glib notions with the world. probably not even a real person.
Rory Allen: “ science deniers will seize on any lack of consistency in the models, or any failure to update them, to discredit climate research.”
Michael Wallace”Oh, and I’ve lost relatives to Holocaust
and this proves Rory Allen’s points to be “ glib notions” – how?
MW to RA: you Poser […] probably not even a real person.
Take that, you probably not even a real person! Your mother was a hamster, and your father smelt of elderberries!
Hmm. I’m not going to be around long enough to see the results of “Operations”, so I choose to have fun doing “Research” and hop I can plant a seed that Operations will eventually be able to turn into something useful long after I’m gone.
Totally agree that operationalization of climate science is needed to enable urgent climate action, similar to what has happened for weather prediction many years ago. Your post neglects to mention C3S (the Copernicus Climate Change Service), which was established precisely for this purpose. Don’t you think that C3S can play a major role in this endeavor?
[Response: Definitely, they were part of the workshop last month, and there are real synergies to be worked out. – gavin]
1) One problem that may arise is if rich people start losing $billions in real estate value when your projections are used by organizations like First Street to project flooding etc. Homeowners in Florida are starting to find it difficult to get home insurance.
https://firststreet.org/
2) In the past the projections were less inciting because they were 30 years in the future and not widely known among the population.
3) Science, of course, should not let people’s financial or economic issues disrupt the search for the truth. However, I think of large models as tools, not evidence . It can be difficult to verify large software programs are correct even with detailed code inspections by review teams–as witness Microsoft’s frequent need to correct security problems in Windows.
4) Climate science problem has been trying to show events are due to climate change when there is such wide variability in the weather. We have had major droughts, floods and hurricanes 100 years in the past although the melting of the Arctic icecap and glaciers are physical evidence, But the danger has been that by the time conclusive physical evidence –convincing to the average voter or jury –arrives it will be too late,
Uninformed New media claiming every event is climate change does not help.
5) I’m stating the obvious to point out that what works in a science conference may not work as well in lawsuits filed by very wealthy parties or in major political attacks. The more useful you become the more some powerful people may hate you. Interesting incentive structure.
Home owners in FL are “starting” to find it difficult? I disagree: W are well beyond the start point.
Ironic that my 2018 UNM dissertation proposal which covered accurate decadal scale hydroclimatological forecasts (based on solar cycles) was rejected because according to the committee, there was no societal value or need for decadal scale forecasts.
Now years later seems like y’all are plagiarizing with prejudice. The fact that you block me is also a concern. I did give an invited talk on my solar forcing research to JPL last September and didn’t see you there.
Gavin noted:
“… in explaining recent temperature anomalies”
Presumptuous to attach an “operational” label to characterizing a process such as natural variability that is still in flux. Where does such a model lie on the spectrum of (1) research-grade (2) prototype (3) pre-operational (4) operational (5) production-grade (6) shrink-wrapped ?
Having worked on everything from shrink-wrapped delivery (engineering software) to production-grade (proprietary semiconductor process control) down to prototype (robotics visualization) and research-grade (scientific modeling), I have found it difficult to get out of the latter category unless one has extreme confidence in the results and the model matches details in observations.
If there is a breakthrough that can be included in a model but includes considerable rework, the research-grade will be most adaptable. In contrast, the maintainers of the operational product will guard their intricate work and belly-ache that it will take too much effort to change.
The caveat in all this is if “explaining recent temperature anomalies” is not important. Then develop and maintain a mean-value operational product that doesn’t pretend to do all the details.
I do not envy someone on a team tasked with developing a product that does not include understanding of the fundamentals.
I’ll provide an example from our POB blog. Enno Peters developed an analysis for LTO production called ShaleProfile. He did all this work because he was curious about how long the shale reserves would last. It was really mean-value analysis that tracked production data and could forecast. It was operational enough that he sold it to a company called Novi Labs several years ago. The reason it worked so well is that at it’s core, it’s just bean-counting, but with a solid foundation and a well-maintained database. Not sure of the status of the original but the outgrowths are using machine learning (which may or may not be marketing hype). https://novilabs.com/news/novi-labs-announces-the-acquisition-of-shaleprofile/
Gavin’s team are adopting my research approach, without attribution is all. My satellite reanalysis based lagged regression approach was successful. I accurately forecast a decadal scaled climate parameter years in advance. I posted prediction well in advance, and published in the peer reviewed Hydrological Sciences Journal, under intense resistance. The reviewers took their time, across 4 calendar years. Gavin and team never cited, but here’s the kicker.
I was suspended from my Ph.D for only one stated reason: the committee determined that decadal climate forecasting was an inappropriate climate research topic.
Now Gavin and team all gung ho. I guess that next they will have to next appropriate my solar forcing work, since that’s the only way to get to accuracy in decadal climate forecasting.
GISS projections were never accurate about the most important climate parameters and patterns, including moisture.
I was accurate though. Wallace HSJ 2019. It was a set of streamflow projections based on lagged correlations to THE SUN. Yet GISS never cites. So long as they are permitted to disregard work like mine (by never citing), they’ll continue to misrepresent the quality of their own forecasts as high.
I guess there will be no engagement here.. That’s a tactic which almost always works, except for now, I just shared solar truths with scores of your colleagues. They seemed a bit concerned, but unlike GISS, they appear to want to develop better decadal scaled forecasts (and I pointed them to a nice solar tool).
Climate scientists should never mention sunspot cycles as a possible contributing factor. It always seems to bring out the conspiratorial-types such as @Mike8Wallace, who, incidentally blocks me on Xitter. Why Wallace and others latch on to sunspots (the 3rd strongest solar cycle) I’m not certain, but I think it has much to do with the uncertainty of the sunspots feeding the FUD of the overall argument.
I continue to look at tidal forcing as an antidote to this sunspot focus. As it is, lunisolar forcing guides so many of the known massive geophysical behaviors that it really needs another look to help explain the unresolved phenomena. Please read this post https://geoenergymath.com/2024/11/10/lunar-torque-controls-all/, and consider the unification it brings to geophysics dynamical behavior. If for nothing else than providing an alternative to the incessant hyping of sunspots as a forcing factor, it’s worth an appraisal.
Hello Paul Pukite, If only you would read and cite literature. That’s part of any authentic scientist’s job. I’ve ALREADY made accurate forecasts using sunspots and made it through peer review. Quite a while ago. Please don’t tell me what climate scientists should or should not do. It just comes across like posing. In fact it appears you don’t even publish in the field.
I don’t recall blocking any real scientists, so if I blocked you, you probably deserved it for being a troll. what’s your handle? Tell you what, get something published in the climate field and I’ll unblock.
Anyway I’ve been blocked by Gavin on X, and I HAVE published. And that alone should be a red flag for any who read this. And X much better for interaction. I’m @Mike8Wallace. Enjoy your echo chamber while you still have time.
MW: Anyway I’ve been blocked by Gavin on X, and I HAVE published. And that alone should be a red flag for any who read this.
BPL: Yes, blocking people who post obnoxious nonsense on a privately owned blog is CENSORSHIP!
MW: I guess that next they will have to next appropriate my solar forcing work, since that’s the only way to get to accuracy in decadal climate forecasting.
BPL: I believe your constant accusations of plagiarism are mistaken. It is possible for people to think of something you have also thought of without them having telepathy or stealing from you. Especially when it is something as vague as “solar forcing.”
Look up plagiarism, intent has nothing to do with it. But bringing it up very important for all who try to advance in science.
And the issue WILL be solar forcing because Gavin, and you, will have to accept facts if you wish to keep working in the field.
The current issue is that I pioneered use of operational data to forecast climate half a decade in advance, accurately. Yes I used Solar cycles but also satellite reanalysis data. For that achievement, I was suspended from Ph.D. program. As I said, only given reason was their view that my work did not add any value. Now Gavin back with my idea, and no attribution.
And although my Ph.D was denied, my paper was published. First successful climate scaled prediction. beats hell out of any GCM. I didn’t see you at my talk to NASA last September. You should pay attention.
MW: I guess that next they will have to next appropriate my solar forcing work, since that’s the only way to get to accuracy in decadal climate forecasting.
BPL: I believe your constant accusations of plagiarism are mistaken. It is possible for people to think of something you have also thought of without them having telepathy or stealing from you. Especially when it is something as vague as “solar forcing.”
Particularly that Gavin’s article is NOT about your (MW’s) “FORECASTS” of the future – but about what has already happened – improving quality of the HISTORICAL data, so the explanation of GMST today, and projections of the future – do not start with their initial inputs for “today” represented by some data being several years old, e.g.:
Gavin: “ both the ECMWF reanalysis (ERA5) and the MERRA2 effort used the CMIP5 forcings from 2008 onwards for their solar forcing. But these fields are the predictions made around 2004 and are now about half a solar cycle out of sync with the real world.”
Incidentally, it seems that CMIP5 was making solar forcing predictions … at least in 2004. You published your work when? 2019?
Finally – Gavin talks about modern models using “the conc. of CO2, other greenhouse gases, air pollution, land surface change, ozone, the sun, volcanoes, irrigation, meltwater etc.”
while you claim to have achieved “ accurate decadal scale hydroclimatological forecasts – based on solar cycles . Correct me if I am wrong – but if you got “accurate forecasts” based on a SINGLE variable (“solar cycles”) – are you saying that ALL other potential climate forcings ARE therefore NOT IMPORTANT at decadal time scale?
And since the solar cycles by their nature are CYCLES, then are you saying that THERE IS NO TREND at the time-scale of decade(s)? How then do you explain: GMST warming of 0.20°C per decade ( ERA5 from 1979 to 2023).
Couldn’t THAT be another reason why Gavin hasn’t attended your talk on your solar forcing research?
I’ll keep it fresh: At JPL in Pasedena two months ago, I also presented on lagged solar correlations to CO2 of all things. They are very high!
And to Ozone.. yep, solar correlation really impressive.
I also noted that Ozone falls from the sky and expresses overland flow, likely a new discovery by the way, never identified by any before. And the current ozone narrative whether in stratosphere or surface, has a lot to do with all of the smog misunderstanding.
I also presented on NASA’s omission of all ocean CO2 upwelling:
Massive and epic upwelling of CO2 dominates the atmosphere. Yet NASA completely eliminated that data from their narrative. (weird too since this was a sounder meeting) I made a nice slide to demonstrate that.
I don’t know if NASA has posted my presentation yet, but they indicated that this would happen. in meantime, I have copies.
Michael Wallace said:
“I don’t know if NASA has posted my presentation yet, but they indicated that this would happen. in meantime, I have copies.”
You have a website, just post it there. Jeez. This isn’t hard..
I’ve posted the highlights on X. The bulk is integrated into a submission for peer review. If only you had gone to the meeting. But looks like you weren’t invited. Even Gavin was missing, but plenty of GISS operatives there anyway, quietly absorbing my work along with the suits from NASA and NOAA to incorporate into new operational paradigm but without any attribution, as usual.
If only
Gavin should talk to RepublicEn before the usual suspects from K Street monopolize the conversation.
Hey Russell: Republicans Target Social Sciences to Curb Ideas They Don’t Like. A “gift” link: IDK if it will work multiple times.
Sub-title: Conservatives in Florida have moved from explosive politics to subtler tactics to uproot liberal “indoctrination” in higher education by removing subjects like sociology from core requirements.
Would this be worth one of your comic lash-ups?
“Science politicized is science betrayed!”
Dear Dr. Schmidt,
I would like to ask if in the workshop that you mentioned in your article, you discussed also options for pilot projects testing implementation of new, yet unexplored approaches, like fluid dynamics combined with sophisticated tidal force analysis as developed by Paul Pukite?
I have no idea how complicated can such an implementation be, however, I think that the sole option to test validity or invalidity of the proposed theory is a test if it indeed helps improving predictions of climate variations like ENSO.
Best regards
Tomáš
Tomas Kalisz: “I have no idea how complicated can such an implementation be”
…. and yet it does not stop you from assigning work to others
“Sealioning is a type of trolling or harassment that consists of pursuing people with relentless requests for evidence , often tangential or previously addressed, while maintaining a pretense of civility and sincerity (“I’m just trying to have a debate”), and feigning ignorance of the subject matter. It may take the form of “incessant, bad-faith invitations to engage in debate” and has been likened to a denial-of-service attack targeted at human beings [that’s not your first, not the tenth, demand toward the moderators of RC that they answer your questions or test you pet theories.
I think that the sole option to test validity or invalidity of the proposed theory is a test
ONLY if the theory is solid and could deliver cost-effective improvements to the work of Schmidt’s group. But whether it is and it could – of all people here – you are the LEAST QUALIFIED to judge – given your fundamental ignorance of the climate sciences, and unwillingness to learn anything that contradicts your overarching “anything, but GHGs” denier narrative.
in re to Piotr, 21 Nov 2024 at 6:50 PM,
https://www.realclimate.org/index.php/archives/2024/11/operationalizing-climate-science/#comment-827192
Hallo Piotr,
Dr. Schmidt reports about efforts to improve the understanding to mechanisms of natural climate variations. Paul Pukite asserts that he published a theory that may become helpful in this respect.
You cannot wonder that plain people like me ask Dr. Schmdt if an implementation of this theory into climate models is being considered and/or how complicated task can it be.
Greetings
Tomáš
Tomas Kalisz: “Dr. Schmidt reports about efforts to improve the understanding to mechanisms of natural climate variations.
No he doesn’t. Had you read his article and not only Paul Pukite response – you would have known that the article is about something completely different:
Gavin: “Our opinion piece though was very focused on one key aspect – the updating of forcing data files, and the standardization of historical extension simulations by the modeling groups.”
So the article is about the rapid availability, quality and standardization of the RECENT input data to be used in various modelling applications. To this end he proposes: e.g. ” a zeroth order estimate of the last year’s data by July the following year. i.e. we should be able to get the 2024 data extension by July 2025. ”
So no – he does NOT “ report about efforts to improve the understanding to mechanisms of natural climate variations., heck, does not even use the word “natural”. You may have been duped into thinking otherwise by Paul Pukite for whom ANY article is a good pretext to promote the importance of his work on “ natural variability“.
Next time – do your homework – read the original article before making demands on its author,
I thought I was reasonably well informed on climate issues, as civilians go, but I confess I have no clue what “operational” means in this context. Has anyone got a cluestick (and/or link) to hand?
He means a shorter term climate focus than 100 years. All of the fearful long term models that GISS promotes cannot be validated in any way. Same for thousands of academics who count on GISS. They all tethered their careers to climate projections that are not falsifiable. Meanwhile, weather in comparison to climate, is ‘operationally’ modeled for a short frame of a few weeks.
That’s one reason why I am raising a concern, call it enlightened self-interest. As a Ph.D student, I deliberately ignored GISS models and worked with operational reanalysis data to make a set of semidecadal forecasts of streamflows in the Southern Rocky Mountains. The projections were accurate. A historic first. So oddly enough, I was fired from my Ph.D. program and my solar forcing accuracy operational-scaled paper never cited. Now after almost a decade, Gavin sings different tune, even while blocking me on X.
It’s important to realize that Solar forcing of climate has been eliminated from the GISS climate narrative. Solar forcing is decadally scaled, Solar forcing was buried under NASA GISS floorboards and now looks like they are telegraphing a move to bring it back, without attribution to the guy who pioneered it, and lost his Ph.D in process. Way more to unwrap, considering that if Solar is right, then greenhouse gases must be completely wrong. I think that is now proven and just a matter of time for it to sink in to establishment.
Michael Wallace says:
That’s a weird way of saying that you couldn’t cut it.
If you really have something significant, I would be the first to support you.
following up with BPL comment: if you believe that Solar Forcing is “vague’, then you have a lot to learn and perhaps to account for. I took a look at one of your modeling papers, so easy to use a model to speculate on imaginary planets. So hard to predict real climate where and when it counts. I managed to achieve that, but I guess you will never do so until GISS follows my lead.
.
Whether water, temperature, winds, ozone, divergence of latent heat, and even CO2, ALL show statistically significant lagged correlations to Solar forcing (Call it whatever you like, I use sunspots and a TSI series at times). Much of this was profiled in my 2019 paper. More was profiled in my presentation to NASA in 2022 and also last Fall, and additional work headed for journal submissions with colleagues from great institutions other than GISS.
Gavin,
You should check with your colleagues in the property insurance industry, and the broader insurance sector, because they have for many years indeed operationalized climate science due to their direct financial exposure.
Insurers rely on climate science to build catastrophe models that predict the likelihood and severity of events like hurricanes, floods, and wildfires. They integrate climate-related risks into policy structures, including exclusions for high-risk areas or variable premiums based on geographic exposure to climate hazards.
Insurers also promote risk mitigation measures, such as improved building codes, flood defenses, and fire-resistant materials, aligning with climate science insights to reduce claims costs, and many insurers are required by regulators to account for long-term climate risks in their solvency assessments and disclosures.
Let’s not forget that the Reinsurers, which provide insurance to insurers, are also heavily invested in climate science utilizing advanced models to manage the aggregated risk portfolios of primary insurers.
Climate science dictates the strategic, while operations guides the tactical execution of the business plan.
Operationalizing Climate Science
17 Nov 2024 by Gavin
The work of climate modelling scientists might be seen as esoteric if it focuses on niche technical issues rather than addressing the broader, pressing problems of climate change. The issue as presented suggests to me a narrow or inward-looking focus, isolated from broader concerns. Focused inwardly means it’s relevant only within the specific group or discipline. In other words Self-referential and/or Insular and not of much public interest.
While obviously relevant to the work activities of modelling scientists I think this issue is ‘a curiosity’, even though it still points in the direction of underlying much more important issues in need of urgent attention — if anything can ever be done to address the global warming and other critical ecological problems and sustainability.
A lack of Operational Data and Science Analysis is in fact not the barrier to effective environmental actions or addressing energy use across the world. It’s Leadership and Governance – or rather a lack of them.
Summary of My General Thoughts
I have often and for a long time now expressed my significant frustration with the perceived lack of overarching authority or governance in the global climate science and policy landscape. My view suggests that the current system is highly fragmented, with critical activities—such as the provision and analysis of climate data—operating without unified leadership or accountability. I argue that:
1. Leaderless Coordination: Organizations like the IPCC and others fail to provide the strong leadership needed to manage these disparate activities effectively. There is no single authority with the legal or societal mandate to ensure cohesive direction.
2. Self-Governance by Scientists: Climate scientists, while experts in their field, often operate independently, making decisions and setting priorities without external oversight, democratic input, or societal checks.
3. Dysfunction in Global Climate Policy: Bodies like the IPCC and UNFCCC are seen as engaging in self-serving or insular processes that do not adequately address the real-world urgency of climate action, leaving global responses fragmented and ineffective.
4. Questioning Existing Frameworks: I question whether institutions like the IPCC, UNFCCC, and the annual COP meetings are still “fit for purpose” in addressing the existential threat posed by climate change.
Expert Commentary and Public Discourse on the Issue
1. Decentralized Climate Governance:
Many experts agree that global climate governance suffers from fragmentation. For instance, researchers in international relations have critiqued the UNFCCC’s inability to enforce binding commitments from its member states, leading to a patchwork of voluntary and inconsistent efforts.
Dr. Michael Oppenheimer, a prominent climate scientist, has noted that while the IPCC provides an invaluable synthesis of science, it lacks mechanisms to compel governments to act on its findings.
2. Criticism of the IPCC and COP Processes:
Scholars like Dr. Roger Pielke Jr. and Dr. William Nordhaus have questioned whether the IPCC’s structure—focusing on consensus rather than actionable policy guidance—is suitable for urgent climate crises.
Critics of the annual COP meetings, including activists like Greta Thunberg, have described these gatherings as “performative” or a “talking shop” that results in little substantive progress.
3. Calls for New Models of Leadership:
Some experts advocate for a centralized global climate authority, akin to the World Health Organization, that could coordinate efforts, enforce commitments, and manage funding. For example, the economist Jeffrey Sachs has proposed a UN-led “Climate Agency” with enforcement capabilities.
Others emphasize the need for regional and national integration, suggesting that coalitions of willing nations or blocs could lead by example.
And-
4. Operationalizing Climate Science:
Gavin Schmidt, the NASA scientist behind the Real Climate blog referenced, has argued for greater integration of scientific operations into decision-making processes, but he acknowledges the challenge of aligning data providers, governments, and policymakers under one framework.
More specifically–If the following is not adequately addressed, whatever the Climate Modelling Scientists like Gavin do with their time and resources is irrelevant–moot.
Is the IPCC Still Fit for Purpose?
The IPCC has been vital in summarizing and disseminating climate science, but its limitations include:
– Consensus Focus: The need for unanimous agreement often dilutes urgent or controversial recommendations.
– Lack of Enforceability: The IPCC issues reports but does not have authority to enforce actions based on its findings.
– Lag in Responsiveness: Its long report cycles mean it cannot address rapidly changing climate challenges with agility.
These issues have led some critics to argue that the IPCC is increasingly obsolete in a world that requires faster, more actionable responses.
Are the UNFCCC and COP Meetings Still Fit for Purpose?
Critiques of the UNFCCC and COP processes center on:
– Lack of Binding Mechanisms: The Paris Agreement relies on voluntary national commitments, which are often insufficient and inconsistently implemented.
– Overemphasis on Negotiation: Annual COPs often focus on political posturing rather than delivering concrete action plans.
– Exclusion of Key Voices: Indigenous groups, youth activists, and developing nations frequently criticize the process for prioritizing the interests of wealthier, more powerful countries.
– Questions abound about the usefulness and accuracy of today’s Economic norms and systems
Some have proposed alternatives, such as regional climate pacts or sector-specific agreements (e.g., for energy or agriculture), as more pragmatic and effective solutions.
Recommendations for a Better Framework
1. Establish a Global Climate Authority: Create a centralized entity with binding authority to direct and enforce climate action.
2. Move from Consensus to Leadership: Shift away from consensus-driven approaches to allow bold leadership by coalitions of willing nations or regions.
3. Integrate Science and Policy: Operationalize climate science by embedding experts directly within decision-making frameworks at all levels of governance.
4. Strengthen Accountability Mechanisms: Require transparent reporting, enforce penalties for non-compliance, and involve civil society in monitoring.
This critique aligns with these growing calls for urgent reform, emphasizing that the current frameworks are inadequate for addressing the scale and urgency of the climate crisis.
Gavin
After reading this post and some of the commentary, it occurred to me that FEMA FIRM Maps, which show incredible amounts of information about flood prone areas, might provide a model for how climate scientists might approach risk assessment in other areas which might be affected by climate change, including drought areas, susceptibility to large scale fires, changes in agricultural use, etc.
FIRM Maps are an important resource because owners, investors, real estate agents, architects, engineers, insurance companies and all levels of government value the information that helps with risk assessment. Nothing in construction is completely risk-free, but this information is vital when making a financial investment.
I think that the reason that insurance companies are pulling out of fire prone areas in the west and flood prone coastal cities in the southeast isn’t because they can’t raise rates in the face of increasing risks brought on by climate change – insurance companies raise rates because of additional risk all the time. The problem is quickly becoming one in which there are no way to quantify the risk with the rapid changes we are experiencing.
Attached is a link to a FIRM Map that I had experience using a few years ago. It’s a good example because it shows so many flood prone areas on the coast, along the river and even inland flooding that affects the coastal plain.
https://msc.fema.gov/portal/search?AddressQuery=waimea%20kauai
Note: It’s not really hard to imagine an update of this map in 20 years with virtually all the elevations changed.